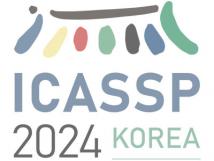
IEEE ICASSP 2024 - IEEE International Conference on Acoustics, Speech and Signal Processing (ICASSP) is the world’s largest and most comprehensive technical conference focused on signal processing and its applications. The IEEE ICASSP 2024 conference will feature world-class presentations by internationally renowned speakers, cutting-edge session topics and provide a fantastic opportunity to network with like-minded professionals from around the world. Visit the website.
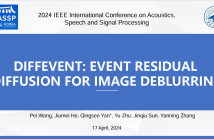
Traditional frame-based cameras inevitably suffer from non-uniform blur in real-world scenarios. Event cameras that record the intensity changes with high temporal resolution provide an effective solution for image deblurring. In this paper, we formulate the event-based image deblurring as an image generation problem by designing diffusion priors for the image and residual. Specifically, we propose an alternative diffusion sampling framework to jointly estimate clear and residual images to ensure the quality of the final result.
- Categories:
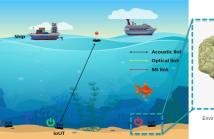
- Read more about Energy Efficient Wake-Up Solution For Largescale Internet Of Underwater Things Networks
- Log in to post comments
Underwater monitoring and exploration have enhanced significantly due to the wide adoption of Internet of Underwater Things (IoUT). However, IoUT implementation is limited by batteries that require frequent replacement, which is costly and unfeasible due to the hostile aquatic environment. Therefore, it is crucial to implement an energy-efficient solution that maximizes the lifetime of IoUT devices, and hence reduce the overall cost of the system.
- Categories:
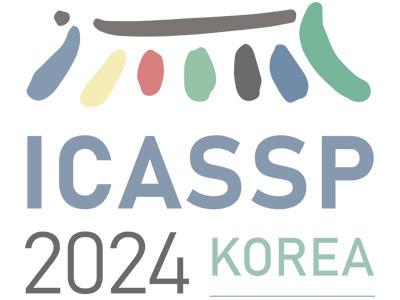
- Read more about Remixing Music for Hearing Aids Using Ensemble of Fine-Tuned Source Separators
- Log in to post comments
This paper introduces our system submission for the Cadenza ICASSP 2024 Grand Challenge, which presents the problem of remixing and enhancing music for hearing aid users. Our system placed first in the challenge, achieving the best average Hearing-Aid Audio Quality Index (HAAQI) score on the evaluation data set. We describe the system, which uses an ensemble of deep learning music source separators that are fine tuned on the challenge data.
- Categories:
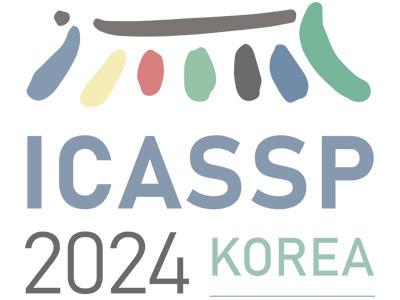
- Read more about Cyclic Misspecified Cramer-Rao Bound for Periodic Parameter Estimation
- Log in to post comments
In many practical parameter estimation problems, the observation model is periodic with respect to the unknown parameters. In these cases, the appropriate estimation criterion is periodic in the parameter space, and cyclic performance bounds should be used. However, existing cyclic performance bounds do not account for the common scenario of model misspecification. The misspecified Cramér-Rao bound (MCRB) provides a lower bound on the mean-squared-error (MSE) for estimation problems under model misspecification. However, the MCRB does not provide a valid bound for periodic problems.
- Categories:
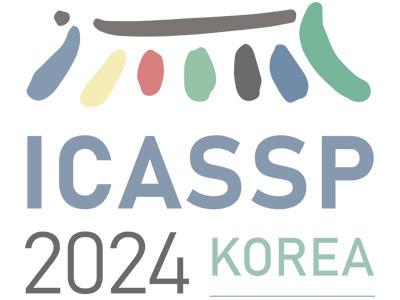
- Read more about SALM: Speech-augmented Language Model with In-context Learning for Speech Recognition and Translation
- 1 comment
- Log in to post comments
We present a novel Speech Augmented Language Model (SALM) with multitask and in-context learning capabilities. SALM comprises a frozen text LLM, a audio encoder, a modality adapter module, and LoRA layers to accommodate speech input and associated task instructions. The unified SALM not only achieves performance on par with task-specific Conformer baselines for Automatic Speech Recognition (ASR) and Speech Translation (AST), but also exhibits zero-shot in-context learning capabilities, demonstrated through keyword-boosting task for ASR and AST.
- Categories:
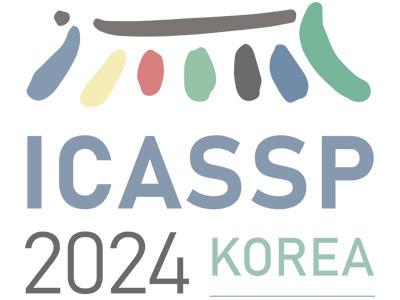
- Read more about SALM: Speech-augmented Language Model with In-context Learning for Speech Recognition and Translation
- Log in to post comments
We present a novel Speech Augmented Language Model (SALM) with multitask and in-context learning capabilities. SALM comprises a frozen text LLM, a audio encoder, a modality adapter module, and LoRA layers to accommodate speech input and associated task instructions. The unified SALM not only achieves performance on par with task-specific Conformer baselines for Automatic Speech Recognition (ASR) and Speech Translation (AST), but also exhibits zero-shot in-context learning capabilities, demonstrated through keyword-boosting task for ASR and AST.
- Categories:
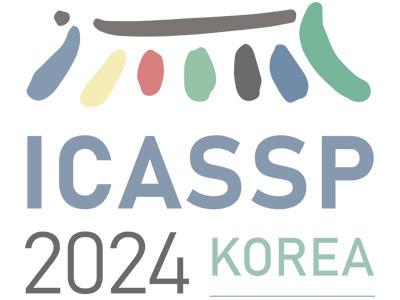
- Read more about How Secure Is the Time-Modulated Array-Enabled OFDM Directional Modulation?
- 1 comment
- Log in to post comments
Time-modulated arrays (TMA) transmitting orthogonal frequency division multiplexing (OFDM) waveforms achieve physical layer security by allowing the signal to reach the legitimate destination undistorted, while making the signal appear scrambled in all other directions. In this paper, we examine how secure the TMA OFDM system is, and show that it is possible for the eavesdropper to defy the scrambling.
- Categories:
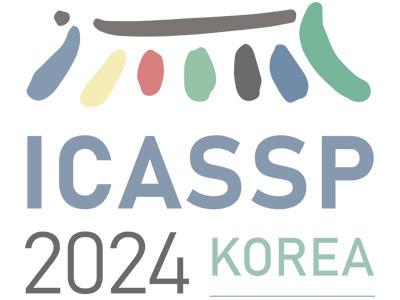
- Read more about END-TO-END SPEECH RECOGNITION CONTEXTUALIZATION WITH LARGE LANGUAGE MODELS
- Log in to post comments
In recent years, Large Language Models (LLMs) have garnered significant attention from the research community due to their exceptional performance and generalization capabilities. In this paper, we introduce a novel method for contextualizing speech recognition models incorporating LLMs. Our approach casts speech recognition as a mixed-modal language modeling task based on a pretrained LLM. We provide audio features, along with optional text tokens for context, to train the system to complete transcriptions in a decoderonly fashion.
- Categories:

- Read more about Presentation of Diffusion-based speech enhancement with a weighted generative-supervised learning loss
- 1 comment
- Log in to post comments
Diffusion-based generative models have recently gained attention in speech enhancement (SE), providing an alternative to conventional supervised methods. These models transform clean speech training samples into Gaussian noise, usually centered on noisy speech, and subsequently learn a parameterized
- Categories:
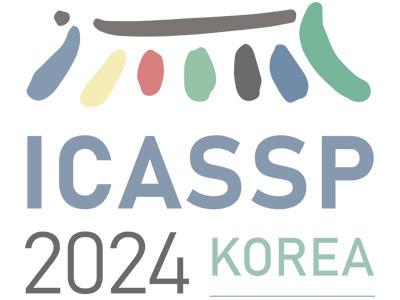
Inference of time-varying data over graphs is of importance in real-world applications such as urban water networks, economics, and brain recordings. It typically relies on identifying a computationally affordable joint spatiotemporal method that can leverage the patterns in the data. While this per se is a challenging task, it becomes even more so when the network comes with uncertainties, which, if not accounted for, can lead to unpredictable consequences.
- Categories: