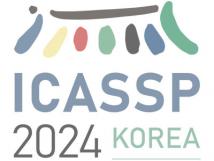
IEEE ICASSP 2024 - IEEE International Conference on Acoustics, Speech and Signal Processing (ICASSP) is the world’s largest and most comprehensive technical conference focused on signal processing and its applications. The IEEE ICASSP 2024 conference will feature world-class presentations by internationally renowned speakers, cutting-edge session topics and provide a fantastic opportunity to network with like-minded professionals from around the world. Visit the website.
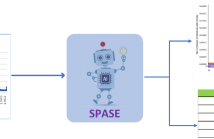
- Read more about SPASE: SPAtial Saliency Explanation for time series models
- 1 comment
- Log in to post comments
We have seen recent advances in the fields of Machine Learning (ML), Deep Learning (DL), and Artificial intelligence (AI) that the models are becoming increasingly complex and large in terms of architecture and parameter size. These complex ML/DL models have beaten the state of the art in most fields of computer science like computer vision, NLP, tabular data prediction and time series forecasting, etc. With the increase in models’ performance, model explainability and interpretability has become essential to explain/justify model outcome, especially for business use cases.
- Categories:
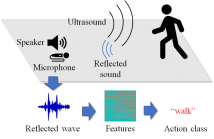
- Read more about HEAR-YOUR-ACTION: HUMAN ACTION RECOGNITION BY ULTRASOUND ACTIVE SENSING
- Log in to post comments
Action recognition is a key technology for many industrial applications. Methods using visual information such as images are very popular. However, privacy issues prevent widespread usage due to the inclusion of private information, such as visible faces and scene backgrounds, which are not necessary for recognizing user action. In this paper, we propose a privacy-preserving action recognition by ultrasound active sensing.
- Categories:
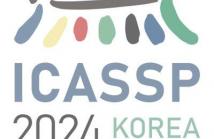
- Read more about Embedded Feature Similarity Optimization with Specific Parameter Initialization for 2D/3D Medical Image Registration
- Log in to post comments
We present a novel deep learning-based framework: Embedded Feature Similarity Optimization with Specific Parameter Initialization (SOPI) for 2D/3D medical image registration which is a most challenging problem due to the difficulty such as dimensional mismatch, heavy computation load and lack of golden evaluation standard. The framework we design includes a parameter specification module to efficiently choose initialization pose parameter and a fine-registration module to align images.
- Categories:
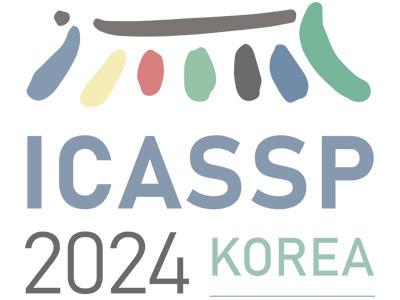
- Read more about EMORED: A DATASET FOR RELATION EXTRACTION IN TEXTS WITH EMOTICONS
- Log in to post comments
Relation extraction (RE) is a vital task within natural language processing. Previous works predominantly focus on extracting relations from plain text. However, with the evolution of communication habits, many individuals employ symbolic representations, e.g. emoticons, to convey nuanced information. This shift in communication prompts a pertinent question: How do emoticons impact the performance of RE models?
- Categories:
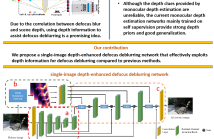
- Read more about EFFICIENT FUSION OF DEPTH INFORMATION FOR DEFOCUS DEBLURRING
- 1 comment
- Log in to post comments
Defocus deblurring is a classic problem in image restoration tasks. The formation of its defocus blur is related to depth. Recently, the use of dual-pixel sensor designed according to depth-disparity characteristics has brought great improvements to the defocus deblurring task. However, the difficulty of real-time acquisition of dual-pixel images brings difficulties to algorithm deployment. This inspires us to remove defocus blur by single image with depth information.
- Categories:
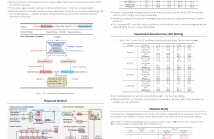
Prompt learning was proposed to solve the problem of inconsistency between the upstream and downstream tasks and has achieved State-Of-The-Art (SOTA) results in various Natural Language Processing (NLP) tasks. However, Relation Extraction (RE) is more complex than other text classification tasks, which makes it more difficult to design a suitable prompt template for each dataset manually. To solve this issue, we propose a Adaptive Prompt Construction method (APC) for relation extraction.
- Categories:
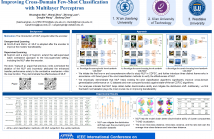
- Read more about Improving Cross-domain Few-shot Classification with Multilayer Perceptron
- Log in to post comments
Cross-domain few-shot classification (CDFSC) is a challenging and tough task due to the significant distribution discrepancies across different domains. To address this challenge, many approaches aim to learn transferable representations. Multilayer perceptron (MLP) has shown its capability to learn transferable representations in various downstream tasks, such as unsupervised image classification and supervised concept generalization. However, its potential in the few-shot settings has yet to be comprehensively explored.
- Categories:
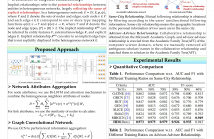
- Read more about HIM: DISCOVERING IMPLICIT RELATIONSHIPS IN HETEROGENEOUS SOCIAL NETWORKS
- Log in to post comments
To date, research on relation mining has typically focused on analyzing explicit relationships between entities, while ignoring the underlying connections between entities, known as implicit relationships. Exploring implicit relationships can reveal more about social dynamics and potential relationships in heterogeneous social networks to better explain complex social behaviors. The research presented in this paper explores implicit relationships discovery methods in the context of heterogeneous social networks.
- Categories:
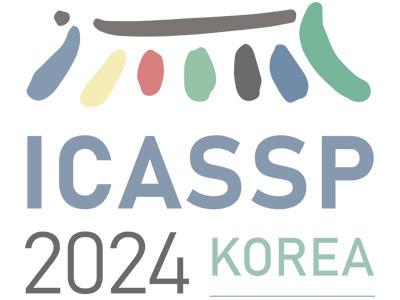
- Read more about INVERTIBLE VOICE CONVERSION WITH PARALLEL DATA
- Log in to post comments
This paper introduces an innovative deep learning framework for parallel voice conversion to mitigate inherent risks associated with such systems. Our approach focuses on developing an invertible model capable of countering potential spoofing threats. Specifically, we present a conversion model that allows for the retrieval of source voices, thereby facilitating the identification of the source speaker. This framework is constructed using a series of invertible modules composed of affine coupling layers to ensure the reversibility of the conversion process.
- Categories:
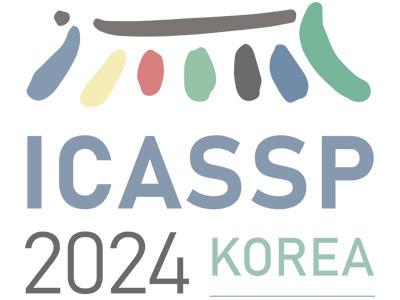
- Read more about Joint Multi-Band DOA Estimation Using Low-Rank Matrix Recovery
- Log in to post comments
To address wideband direction of arrival (DOA) estimation problems, this paper proposes a gridless and covariance-free joint multi-band (JMB) DOA estimation method using low-rank matrix recovery. In contrast with subspace methods and sparse array-based methods, a unified frequency grid is established based on the concept of the greatest common divisor (GCD) to solve the nonlinearity of steering matrices from multiple frequencies. With the unified frequency grid, a low-rank master matrix is formed as a combination of the truncated Hankel matrices from different subbands and snapshots.
- Categories: