Documents
Poster
MossFormer2: Combining Transformer and RNN-Free Recurrent Network for Enhanced Time-Domain Monaural Speech Separation
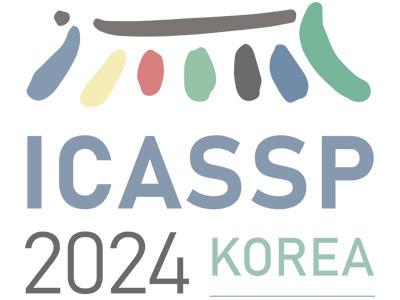
- Citation Author(s):
- Submitted by:
- Shengkui Zhao
- Last updated:
- 14 April 2024 - 3:34am
- Document Type:
- Poster
- Document Year:
- 2024
- Event:
- Presenters:
- Shengkui.zhao
- Paper Code:
- SLP-P37.4
- Categories:
- Log in to post comments
Our previously proposed MossFormer has achieved promising performance in monaural speech separation. However, it predominantly adopts a self-attention-based MossFormer module, which tends to emphasize longer-range, coarser-scale dependencies, with a deficiency in effectively modelling finer-scale recurrent patterns. In this paper, we introduce a novel hybrid model that provides the capabilities to model both long-range, coarse-scale dependencies and fine-scale recurrent patterns by integrating a recurrent module into the MossFormer framework. Instead of applying the recurrent neural networks (RNNs) that use traditional recurrent connections, we present a recurrent module based on a feedforward sequential memory network (FSMN), which is considered "RNN-free" recurrent network due to the ability to capture recurrent patterns without using recurrent connections. Our recurrent module mainly comprises an enhanced dilated FSMN block by using gated convolutional units (GCU) and dense connections. In addition, a bottleneck layer and an output layer are also added for controlling information flow. The recurrent module relies on linear projections and convolutions for seamless, parallel processing of the entire sequence. The integrated MossFormer2 hybrid model demonstrates remarkable enhancements over MossFormer and surpasses other state-of-the-art methods in WSJ0-2/3mix, Libri2Mix, and WHAM!/WHAMR! benchmarks. Our source code is integrated into SpeechBrain toolkit and is also released at https://github.com/alibabasglab/MossFormer2.
Comments
This is our ICASSP 2024 paper
This is our ICASSP 2024 paper work for monaural speech separation. Thank you.