Documents
Presentation Slides
THE ROYALFLUSH SYSTEM OF SPEECH RECOGNITION FOR M2MET CHALLENGE
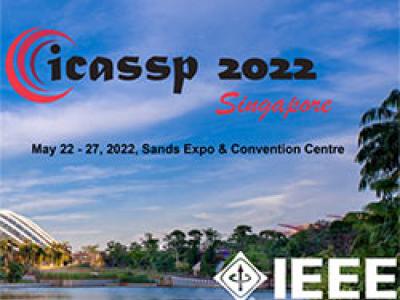
- Citation Author(s):
- Submitted by:
- Shuaishuai Ye
- Last updated:
- 4 May 2022 - 9:33pm
- Document Type:
- Presentation Slides
- Document Year:
- 2022
- Event:
- Presenters:
- Shuaishuai Ye
- Paper Code:
- CHAL-3.6
- Categories:
- Log in to post comments
This paper describes our RoyalFlush system for the track of multi-speaker automatic speech recognition (ASR) in the M2MeT challenge. We adopted the serialized output training (SOT) based multi-speakers ASR system with large-scale simulation data. Firstly, we investigated a set of front-end methods, including multi-channel weighted predicted error (WPE), beamforming, speech separation, speech enhancement and so on, to process training, validation and test sets. But we only selected WPE and beamforming as our frontend methods according to their experimental results. Secondly, we made great efforts in the data augmentation for multi-speaker ASR, mainly including adding noise and reverberation, overlapped speech simulation, multi-channel speech simulation, speed perturbation, front-end processing, and so on, which brought us a great performance improvement. Finally, in order to make full use of the performance complementary of different model architecture, we trained the standard conformer based joint CTC/Attention (Conformer) and U2++ ASR model with a bidirectional attention decoder, a modification of Conformer, to fuse their results. Comparing with the official baseline system, our system got a 12.22% absolute Character Error Rate (CER) reduction on the validation set and 12.11% on the test set.