Documents
Whitepaper
SCENE TEXT RECOGNITION MODELS EXPLAINABILITY USING LOCAL FEATURES
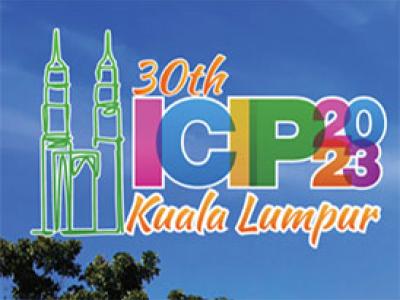
- DOI:
- 10.60864/p4aw-we28
- Citation Author(s):
- Submitted by:
- Mark Vincent Ty
- Last updated:
- 17 November 2023 - 12:05pm
- Document Type:
- Whitepaper
- Event:
- Presenters:
- Mark Vincent A. Ty
- Categories:
- Log in to post comments
Explainable AI (XAI) is the study on how humans can be able to understand the cause of a model’s prediction. In this work, the problem of interest is Scene Text Recognition (STR) Explainability, using XAI to understand the cause of an STR model’s prediction. Recent XAI literatures on STR only provide a simple analysis and do not fully explore other XAI
methods. In this study, we specifically work on data explainability frameworks, called attribution-based methods, that explains the important parts of an input data in deep learning models. However, integrating them into STR produces inconsistent and ineffective explanations, because they only explain the model in the global context. To solve this problem, we
propose a new method, STRExp, to take into consideration the local explanations, i.e. the individual character prediction explanations. This is then benchmarked across different
attribution-based methods on different STR datasets and evaluated across different STR models.